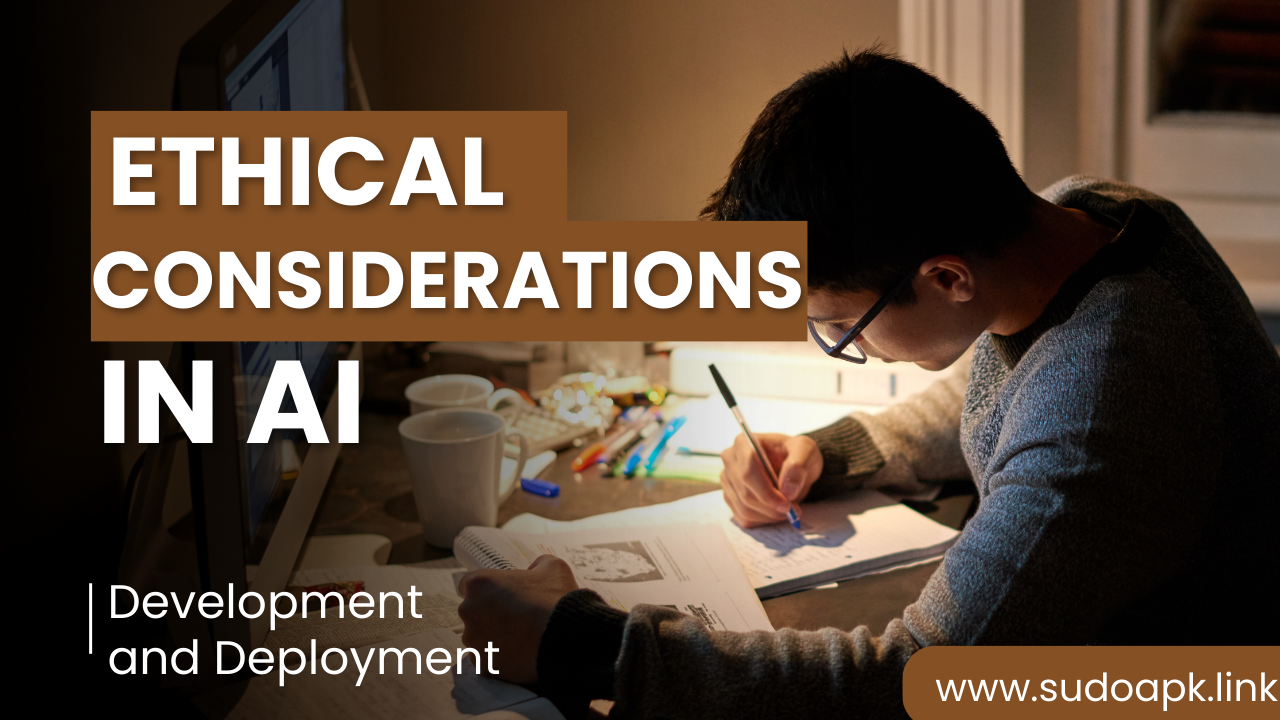
Ethical Considerations in AI Development and Deployment
Artificial Intelligence (AI) systems are being rapidly adopted across industries, enabling breakthroughs in areas like autonomous vehicles, precision medicine, and smart automation. However, as AI becomes more powerful and ubiquitous, we need to carefully assess its integration into society's core systems and address rising concerns around ethics and responsibility.
AI developers and companies deploying AI solutions have an obligation to examine the technology's potential harms early on and institute safeguards to align systems with moral values like trust, transparency, bias prevention and accountability. In this article, we will explore leading frameworks and practical strategies to build ethics into AI by design while deploying responsibly.
Before looking at solutions, we need to understand the central issues that create ethical dilemmas stemming from AI systems:
Many advanced AI techniques like deep learning are complex black boxes with billions of parameters encoding pattern recognition capabilities. Their opacity makes explaining specific predictions and auditing forissues like bias difficult. This harms accountability and trust.
AI models trained on human-created historical datasets often inherit and amplify societal biases around race, gender and culture leading to unfairness. Though unintentional, this could worsen discrimination against minorities.
Over-reliance on AI for high-stakes decisions like parole approval, insurance pricing, hiring and financial trading could amplify risks if systems make incorrect predictions or overlook contextual factors through narrowness. The downstream impacts on people's lives warrant caution.
Advanced systems like autonomous weapons built without safeguards on acceptable behavior risk causing inadvertent harm that violates human ethics. Similarly, neural networks amplifying toxic content online erode social cohesion. AI should align with moral values.
Vast data collection, predictive profiling and behavioral micro-targeting by AI systems, if left unchecked, could seriously undermine personal privacy and human agency. User consent, transparency and oversight mechanisms are essential.
By acknowledging these risks upfront, developers can adopt remedies throughout the machine learning pipeline to make AI trustworthy. Let's analyze leading frameworks and best practices that constitute ethical AI design.
Independent bodies like the IEEE and governments have proposed ethical frameworks consisting of principles that AI systems should demonstrate and processes which support those principles. Let's examine prominent guidelines:
This extensive framework require AI systems to realize:
Human agency and oversight so people can make informed autonomous decisions instead of reliance on prescriptive systems removing user control.
Technical robustness and safety through adequate and secure integration into environments by analyzing risk factors thoroughly.
Privacy and data governance via data minimization, encryption, access control and opt-in policies protecting user privacy.
Transparency to explain system capabilities, limitations and decisions through documentation and communication.
Diversity, non discrimination and fairness by ensuring data and models account for diversity, regularly auditing and patching biases.
Environmental wellbeing through energy efficiency, renewable integration and measuring sustainability impacts over the AI system's full lifecycle.
Accountability via mechanisms to measure, document and remedy adverse impacts stemming from AI systems so responsibility can be upheld.
This set of process-based standards guides technologists on prioritizing ethical considerations during all stages of conception, design, development and deployment of AI solutions via practices like:
Such frameworks offer comprehensive guidance. Complementing principles with tools for specific issues is vital too. Let's analyze some areas:
Rooting out biases requires auditing datasets and intermediate model representations using bias testing suites like IBM's AI Fairness 360, testing model performance across user subgroups and tweaking data/algorithms until fairness metrics converge.
Using model-agnostic interpretation methods like LIME and SHAP to explain model local predictions or employing prototype networks where features map to interpretable representations boosts transparency.
Assessing model performance on perturbed test inputs reflecting bad faith attacks and strengthening architectures accordingly improves reliability and safety. Adversarial rotations familarize models with challenging edge cases.
Using differential privacy, federated learning, homomorphic encryption and trusted hardware to train models without accessing raw data preserves privacy. Decentralized identity tools like eager-id verify consent in data flows.
By combining principles with cutting-edge techniques, we can develop ethical and responsible AI systems. Now let's shift our focus to deployment.
For companies operationalizing AI, responsible deployment is crucial alongside design. Organizations need to evaluate when risks outweigh benefits before deploying AI while scaling gradually and monitoring for adherence with ethics policies. Common strategies include:
Releasing AI tools slowly after safety checks lets deployers gather user feedback, remedy issues and build trust before system-wide adoption. Policymakers use regulatory sandboxes on fintech for the same reason.
Identifying use cases likely to cause material harm via bias amplification or judgment errors needs balanced thinking across disciplines. Domain experts curb limitations in technologists' foresight. Diverse perspectives allow holistic risk analysis.
Check mechanisms like bias monitors, user complaints review, approval processes for high-risk predictions and regular ethical audits enable continuous assurance that AI systems act responsibly after deployment in complex open environments.
Governments, civil society, businesses and technology leaders need to collectively deliberate policies guiding AI deployment through public consultations. Being proactive and open fosters shared responsibility.
Overall, a cocktail of good practices across assessment, community participation, transparency and oversight helps mitigate emerging issues.
With advanced systems reaching human-comparable capabilities, discussing technology regulation alone cannot address AI's broad impacts. Rethinking incentives, updating laws and social contracts to distribute prosperity, easing worker transitions and nurturing humanism become vital too.
Solutions need multidimensional thinking spanning ethics, psychology, economics, welfare and spirituality. Technology leaders acknowledging moral obligations, companies prioritizing people's wellbeing over profits and policymakers shaping conditions for shared thriving can guide AI responsibly.
While technical safeguards engage short-term risks, encouraging altruism and social justice counter technology's dehumanizing impacts, restoring human dignity and purpose. With collective wisdom and compassion, we can build a truly empowering future with AI.
Ethical AI refers to machine learning systems aligned with moral values like trust, transparency, privacy, non-discrimination and accountability through design practices like value-based assessments, bias testing, rights-preserving data usage, explainability and AI safety techniques.
Adopting auditable model reporting, regular bias monitoring, external algorithmic audits, subjecting high-risk models to ethics approval processes and enabling citizens to file AI grievance complaints help showcase accountability and transparency.
Spheres like criminal justice, healthcare, employment and education where AI directly impacts human wellbeing and civil rights require extra diligence around bias testing, explainability and conservatism before integrating algorithmic systems.
It refers to developing policy, regulations, incentives and deployment norms for emerging technology through discussion between governments, businesses, experts and citizens. Bottom-up insights allow balanced, evidence-based and rights-respecting governance.
Using model interpretation methods, documenting data sources, metrics optimized for, assumptions and use case constraints in memos, disclosing model versions actively used and enabling user-friendly interfaces to query models for explanations improves transparency.
In summary, instilling ethics into AI throughout the system lifecycle while adopting responsible deployment strategies can help unlock its benefits for good while improving trust and acceptance. With sound frameworks now emerging, translating principles diligently into practice remains key.
Popular articles
Dec 31, 2023 07:04 AM
Jan 06, 2024 06:56 AM
Dec 31, 2023 06:48 AM
Dec 31, 2023 07:22 AM
Jan 01, 2024 09:54 PM
Comments (0)