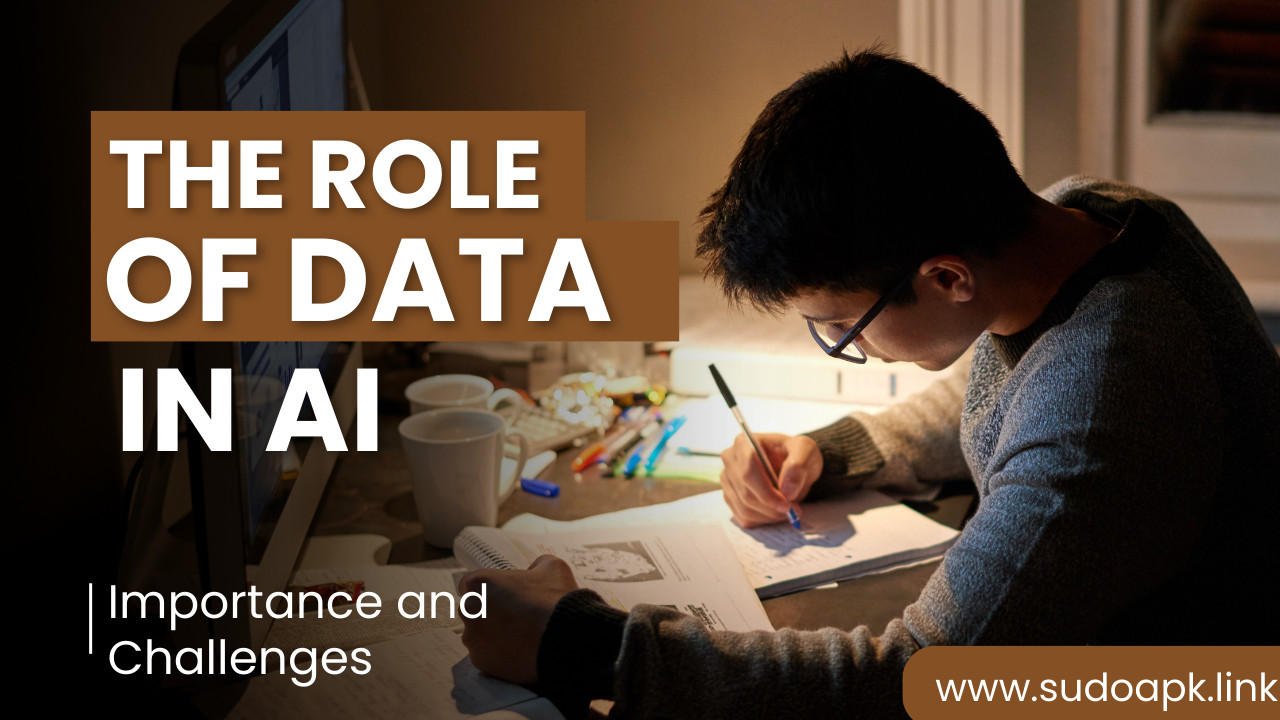
The Role of Data in AI: Importance and Challenges
Behind artificial intelligence’s rapid ascent permeating across industries sits a pivotal driver fueling capability advancement: Data. Vast datasets enable machine learning algorithms to derive meaningful patterns lifting analytical proficiency beyond previous constraints.
Yet data reliance accompanies formidable challenges around addressing real-world complexity, handling outliers and uncertainty, ensuring privacy and avoiding unintended bias. Examining the symbiotic data relationship underpinning AI illuminates the landscape of progress made alongside pitfalls requiring diligent navigation.
Data represents the lifeblood flowing through modern AI systems - the oxygen sustaining measurable progress across automated applications benefiting organizations worldwide. Processing datasets unveils otherwise obscured trends machines can learn from to excel at specialized tasks.
Machine learning qualifies as the workhorse driving contemporary AI advancement by dynamically enhancing system behaviors analyzing patterns within data rather than explicitly programmed rules. Powerful ML models like deep neural networks uncover multivariate correlations between input datasets and target variables to predict outcomes, group similarities, detect anomalies and generate insights without traditional coding.
Sophisticated model architectures stacking algorithmic layers test hierarchical data representations across training rounds gradually optimizing predictive prowess. Access to large, high-quality datasets proves essential “experiencing” enough informative examples for neural networks to reliably interpret signatures within unfamiliar data.
Think facial recognition models gaining increased discrimination distinguishing subtle physiological facial details analyzing millions of images spanning global demographic diversity. Or natural language processing engines absorbing linguistic nuance digesting immense text corpuses learning contextual word meanings and grammatical intricacy.
The promise of AI directly correlates with available data volume and variety feeding machine learning processes.
Furthermore, complex datasets often sprawl vastly beyond reasonable human analysis capabilities across overwhelms of scale. Individual physicians may observe thousands of patients over careers while deep neural networks ingest clinical records from millions of cases spanning decades recognizing previously unrealized predictors elevating diagnostic accuracy. Hedge fund managers weigh hundreds of securities as algorithms parse millions of filings, patents and financial data points informing smarter investment decisions.
Processing such muscular datasets generates insights impossible manually. This data-centered advantage allows artificial intelligence unlocking new realms of operational efficiency and decision intelligence - key priorities across modern enterprises.
Of course, high-performing machine learning models do not run on hypothetical data. Constructing institutional repositories providing structured access to vast information requires significant investment:
Most organizations already capture troves of data generated through daily operations - from customer relationship management systems tracking sales interactions to operational sensors monitoring production machinery vibrations to retail point-of-sale systems containing purchase records. Concentrating this diffuse enterprise data into standardized lakes makes information accessible for analytics. Establishing rigorous data governance protocols ensures quality control supporting business metrics.
Many scientific institutions and governments openly publish immense datasets for research purposes. For example, genomic sequences, biomedical research references, electronic health records or climate sensor readings. While public data conditions unknown factors absent internal control, the quantity of real-world examples trained machine learning algorithms on proves incredibly useful building models - especially earlier in AI adoption journeys with limited internal data then validated against proprietary datasets.
Also consider purchasing access to premium commercial datasets like credit reports, mobile location trails or satellite imagery where needed. For niche needs with little open data available, licensing data streams generates requisite training examples developing customized ML solutions. While buying data inflates costs, the accuracy return on investment often justifies expenses long-term.
Emerging techniques also synthesize artificial data mimicking real-world statistical properties when genuine datasets remain sparse due to confidentiality restrictions or rare occurrences. Simulated data augments training set diversity bolstering model resilience. Combine synthesized data with some labelled examples to render realistic fakes the AI learns from before assessing performance on real samples.
Any data with meaningful signal - or even creatively manufactured data - fuels better AI to a point. But diversity and volume are key.
Feeding raw data dumps directly into algorithms unfortunately yields lackluster insights. Real-world data requires careful preprocessing ensuring only meaningful, structured signals migrate into machine learning model training.
Many ML algorithms learn correlations between input data points and target variables - like connecting symptoms to diagnosed conditions or travel profiles to flight delays. Humans must manually label thousands of example pairs for algorithms to learn from, which proves resource intensive at scale. Crowdsourcing labeling needs through external workforce networks helps expedite content tagging feeding supervised algorithms.
Real-world data tends to demonstrate messiness from missing fields to inconsistencies needing reconciliation to interpret appropriately. Data scientists devote significant efforts “wrangling” information into reliable structures and consistent formats ensuring data integrity training accurate models. Integrating SQL queries, excel transformations, scripts in Python/R and other steps quality checks data flowing into downstream analytics.
Raw data like pixel values or lengthy text necessitate mathematical transformations extracting meaningful numerical features optimized recognizing patterns for particular problems. Condensing descriptive elements into vector inputs demands nuanced domain experience. Better features NOTICEABLY improve model performance.
The journey progressing from dispersed, disorganized business data towards high-quality cleanly preprocessed datasets demands heavy lifting - but pays exponential dividends reaching accurate AI.
Despite remarkable machine learning breakthroughs academically published demonstrating superhuman proficiency on various tasks using clean benchmark datasets - comparable methods often degrade substantially given raw real-world data. The considerable cavern between controlled lab performance and noisy industrial environments emphasizes air pockets requiring creative ingenuity progressing AI from research to practice.
Even after exhaustive data cleaning efforts, real systems generate imperfect data affording unavoidable uncertainty dealing with edge cases absent from homogeneous controlled research environments. Sensor malfunctions recording erroneous instrumentation data, supply chain inventory gaps from shipment delays and users providing ambiguous search queries demonstrate unavoidable realities learning algorithms must smoothly handle.
Furthermore, past statistical regularities may warp over time as populations and behaviors evolve dynamically meaning insights accurate historically require re-validation against contemporary observations. Consumer psychographics, clinical diagnostics, equipment failure modes and financial risk profiles operate as moving targets resisting stationary modeling. Achieving acceptable performance demands recognizing distribution shifts and adaptable retraining mechanisms responding to change.
Well-annotated datasets prime supervised ML algorithms accurately associating inputs with target variables powering classification use cases. But manual labeling limits scaling across more open-ended passive learning frontiers like anomaly detection or video analytics requiring unsupervised methods inferring intrinsic data structures without explicit human guidance. Self-supervised approaches granting AI abilities for autonomous labeling and representation also show early promise.
While surmountable through diligence and creativity leveraging hybrid techniques - the considerable chasm separating controlled academic trials from industrial operational data hinders direct transplantation of published methods. Coaxing reliability honing approaches against edge cases and uncertainty makes the difference implementing AI successfully.
Beyond purely statistical challenges taming data flows optimizing ML proficiency, ethical considerations around privacy, bias and control also demand attention architecting solutions responsibly.
AI systems ingesting swaths of personal data - especially in sensitive categories like healthcare or finance - assume accountability protecting individuals from exposure breaches or misuse. Anonymizing data, restricting access and clearly communicating intended model usage build trusted data relationships respecting user rights. Leaders continually assess privacy risks as capabilities advance.
While accidents of history pervade human culture, AI offers opportunity correcting long-standing imbalances around factors like gender, ethnicity and age manifest through data. But models often implicitly perpetuate discrimination through blind correlation rather than causation. Teams preemptively search for problematic bias proactively choosing equitable alternatives benefitting all people justly through inclusive design.
Impenetrable black-box ML models frustrate human understanding of model logic - especially impactful decisions allocating loans or judging risk profiles meriting transparency. Architecting intrinsically interpretable models, reverse engineering explanations of advanced systems and allowing interactive queries of reasoning uplift open accountability. Keeping humans looped in assessing model rationales maintains responsible oversight.
Cross collaborations between data scientists, domain experts and ethicists guide the safe and thoughtful data practice underpinning reliable AI.
Moving ahead, expect data demands growing in lockstep catalyzing increasingly powerful AI capabilities across global enterprise. But simply amassing data volume introduces vulnerabilities without thoughtful design. Prioritizing pipelines ensuring integrity - both technically and ethically - unlocks sustainable next-generation solutions benefiting business and society.
Ongoing advances collecting, consolidating and structuring exponentially more enterprise operations data into accessible formats allows training ever more discerning machine learning regimes. Manufacturing plants instrument thousands more IoT sensors capturing equipment telemetry. Healthcare networks share orders of magnitude more patient health records and imaging scans via cloud archives. Financial institutions store exploded transaction histories and risk profile metrics in data lakes. Consolidated data means better-informed AI.
Synthesizing realistic artificial data supercharging model development continues maturing - saving costs manual labeling or external data licensing while preserving confidentiality safeguarding sensitive attributes. Perfecting generative algorithms producing tightly controlled synthetic data matching target distributions facilitates customizable training sets without acquisitions.
And centralized data management platforms provide expanded visibility monitoring changing statistical drift over time and instituting standardized governance policies ensuring models perform reliably and ethically across the enterprise. Clean data and responsible practices cement organizational trust in AI.
While data represents the lifeblood flowing through AI, thoughtful design ensures healthy circulation regulating volume and velocity preventing congestion or leakage channeling capabilities most potently. Developing a sound data foundation sets trajectory soaring through operational maturation synthesizing, governing and continuously supplying machine learning engines.
How much data is required for AI?
No universal rule exists - data demands correspond to complexity spanning model architecture, problem ambiguity and performance goals. But typically thousands to millions of quality examples minimize uncertainty.
What kinds of data can fuel AI systems?
All formats show potential – structured tables, unstructured text/media, timeseries streams, graph networks, etc. Feature engineering extracts informative numerical representations for algorithmic consumption.
What are some leading public datasets for AI research?
Many scientific and government groups publish immense datasets around genomic sequences, particle physics readings, biomedical research, climate science, economics, mapping imagery and more.
How do you ensure data quality feeding accurate AI?
Careful verification, cleaning, preprocessing, labeling and transformations structure reliable data for machine learning. And monitoring changing statistical distributions spots unwanted drift minimizing technical debt.
Who owns data rights regarding AI usage?
Individual privacy and enterprise policies govern appropriate data usage. But typically anonymous analytics and insights separate from personal identifiers allow models benefiting stakeholders broadly.
In conclusion, high-quality datasets supply the crucial fuel driving artificial intelligence advancements today through supervised, unsupervised and reinforcement learning regimes unlocking decision intelligence and automated optimization at unprecedented scales. Yet real-world data contains inherent messiness demanding diligent wrangling guaranteeing model integrity. And thoughtful data design adhering to ethical priorities around privacy, fairness and control begets trust in AI translating across organizations and benefiting lives responsibly. Pursuing reliable data pipelines ushers the continued proliferation of artificial intelligence across global business and society at large in the years ahead.
Popular articles
Dec 31, 2023 07:04 AM
Jan 06, 2024 06:56 AM
Dec 31, 2023 06:48 AM
Jan 01, 2024 09:54 PM
Dec 31, 2023 07:22 AM
Comments (0)